Large science data repositories store not only datasets, but also metadata describing the datasets. Datasets in these repositories are often called “big data,” whereas the metadata describing the datasets can be considered as big metadata. Research that uses such big metadata as the data source can be labeled as big metadata analytics. Since 2013 our research team has been investigating the research collaboration networks using big metadata in GenBank, a data repository hosted at the National Center for Biotechnology Information (NCBI) for curated genetic sequences.
Collaboration Capacity and Scientific Capacity
Two concepts are key to understanding the study. One is collaboration capacity, a concept used to represent the size and dynamics of an individual’s or group’s collaboration networks in relation to their productivity and innovative discoveries, and the other is scientific capacity, the aggregation of the knowledge, skills, abilities, and technical facilities of individual scientists (referred to as Scientific and Technical (S&T) Human Capital), as well as their networks of collaborative relationships. Collaboration capacity is considered as a proxy of scientific capacity in our study.
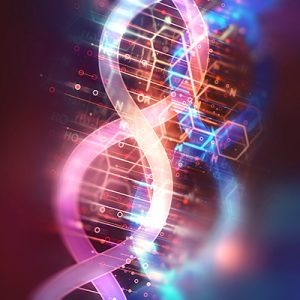
GenBank is the National Institute of Health’s genetic sequence database, an annotated collection of all publicly available DNA sequences.
In cyberinfrastructure-enabled data-intensive science, the size of data submission networks, distribution patterns of such networks, core and peripheral positions of nodes, as well as temporal and taxonomic features can all be used to measure collaboration capacity. Our data show that from 1994 – 2012, the size and shape of data submissions networks evolved from a publication-centric to data-intensive state, in which process the size of giant component steadily increased and almost doubled. While “super-hubs” remained consistently throughout the time period, the data submissions networks had been increasingly branching out. This trend provides evidence for explaining the decrease in clustering coefficient. As a result, the number of data submissions surpassed that of publications by 1997, and has since been in an upward trend.
Another phenomenon we observed from the data was that, while not all authors in data submission networks were in the publication networks, the average ratio of submissions to publications also appeared to be on an upward trend, with a sharp increase from less than one in 1994 to 4.01 around 2005, which was perhaps a turning point for microbiology to become a data-intensive science.
The changes in the ratio of data submissions to publication raise further questions for future research: to what extent data submission networks accelerated and/or facilitated the creation of new knowledge as represented by publications and patents? More broadly, how have data-intensive biology impacted the emergence and evolution of new research areas such as precision medicine? The ratio of submission to publication will be a metric worth further analysis and development for assessing the impact of cyberinfrastructure-enabled data-intensive science.
The big metadata analytics project produced statistical characteristics of research networks and visualized network characteristics through data tables, power law graphs, degree distribution charts, and network structures by year, which offer an evolutionary view of the collaboration networks in data submissions and publications. These results led to the development of a metric framework for assessing the impact of collaboration capacity on the scientific capacity and the role of collaboration capacity in accelerating or slowing down knowledge diffusion.
The Challenge of Messy Data
Our experience proved that big metadata is messy and not well-structured. As such it is extremely challenging to process and transform them into computable structures and formats. We deployed a wide variety of approaches and methods to ensure the quality of data. Based on the approaches and methods used, we generalized a methodological framework consisting of conceptual and computational workflows and collaborative documentation for future big metadata analytics projects.
This project used a novel data source – the metadata from an international data repository GenBank – to study collaboration network structures and dynamics. Examining genetic sequence data submissions and associated publications side by side provides a new perspective on how cyberinfrastructure-enabled collaboration networks have contributed to the advances of data-intensive biology. The resulting datasets made it possible for future research to incorporate other data sources (e.g., funding, patents) to perform data-driven science policy research.